The First euPOLIS Research Papers on major NBS Events
The euPOLIS team has recently submitted research documents and analyses to some of the most significant NBS-dedicated events and conferences, such as the PETRA Virtual Conference held June 29th to July 1st, and NIDS2021 which will take place from September 30th to October 1st, 2021.
The papers are the results of a year-long effort of the team of contributors made of the NTUA experts: Ioannis Kavouras, Eftychios Protopapadakis, Maria Kaselimi, Emmanuel Sardis, and Nikolaos Doulamis.
Machine Learning Tools to Assess the Impact of COVID-19 Civil Measures in Atmospheric Pollution
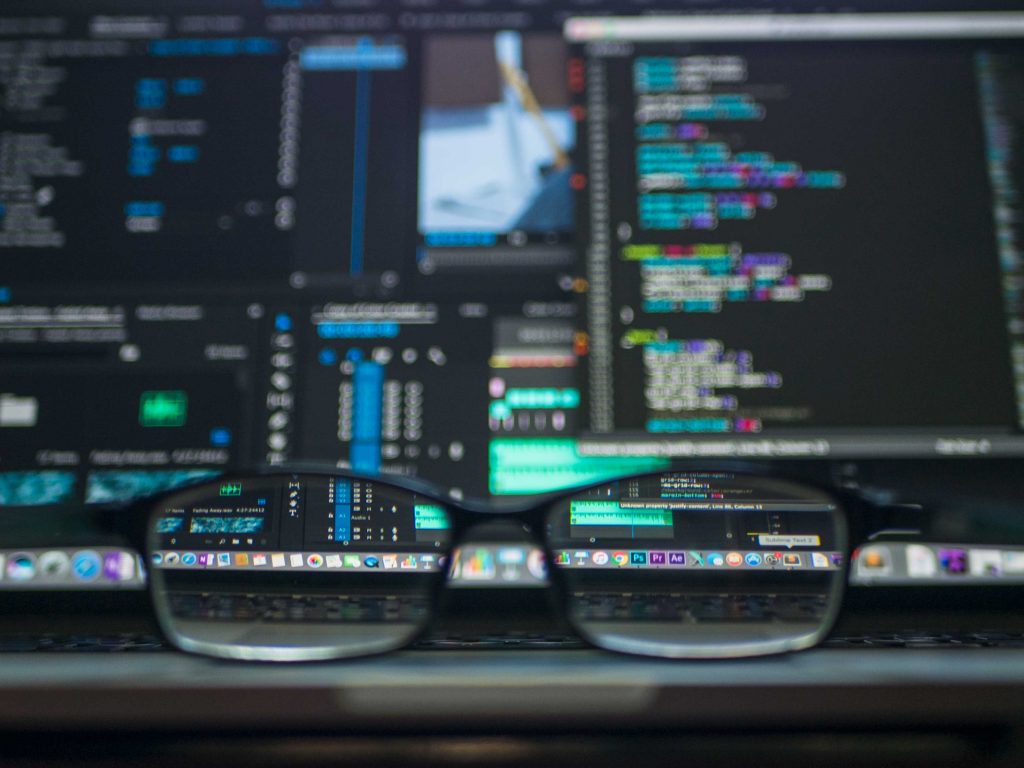
This paper analyses how specific COvID 19 mitigation strategies (e.g. International Travel Controls, Cancellation of Public Events) affect the air quality level in different countries.
In January 2020, a new virus, named SARS-COV-2, was identified and announced to the public; in March the World Health Organization (WHO) declared a worldwide pandemic. To reduce the transmissibility of the new virus, the local authorities, worldwide, introduced a series of measures to flatten the curve. Many of the measures included some form of lockdown and movement restrictions. This unique coordinated worldwide reaction created an opportunity for researching the effects of low traffic on air quality. This research is the relation between the COVID-19 measures and the Air Quality Index (AQI), using four pollutant gases (CO, O3, NO2, SO2). Also, a variety of machine learning tools was used (DNN, DTR, K-NN, Lasso, LReg, MAdam, MGBR, RFR, Ridge) to estimate the accuracy of each method in the prediction of the concentration for each gas one week later. The results showed that after the strict COVID-19 restriction measures the concentration of each pollutant gas reduced rapidly and increased again after the relaxation of lockdown measures. Finally, in cases like Australia, where the measures weren’t as strict as other countries, no improvement was observed.
Assessing the Lockdown Effects on Air Quality during COVID-19 Era
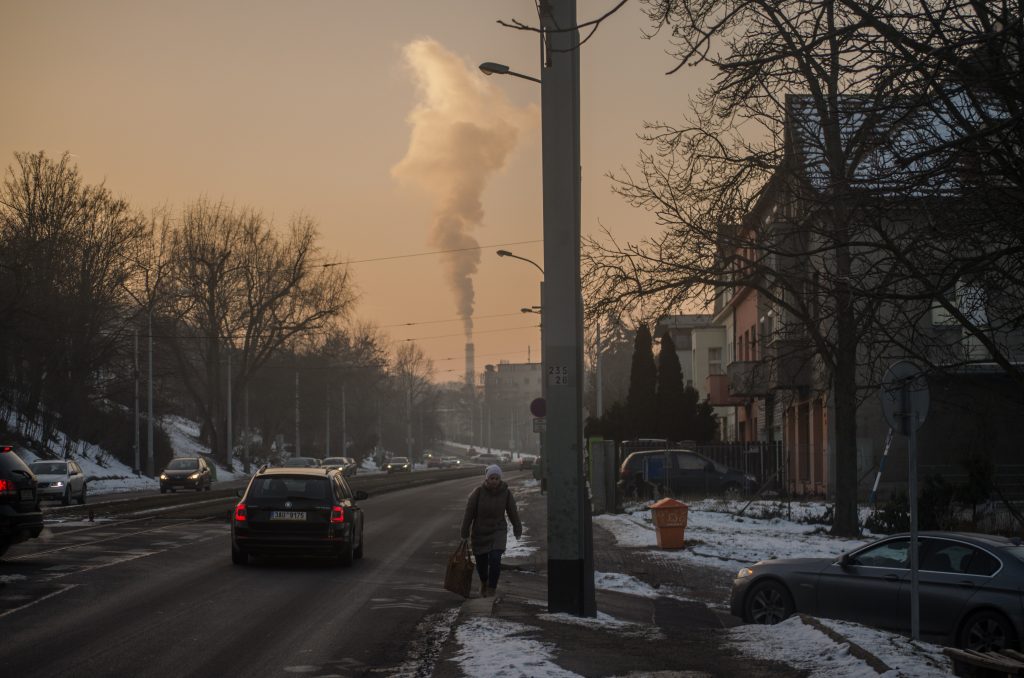
This is a more detailed work that analyses further two things:
-how each of the specific prevention measures is related to each of the pollutant factor levels, and
-to what extent we could estimate (forecast) the trend of each pollutant level, when using as input the current level, plus the current prevention measures
In this work, the researchers indicated that a weak correlation between the COVID-19 and the behavior of the four most dangerous pollutant gases exists. To prove this correlation they performed a series of tests using Pearson and Dynamic Time Warping techniques for each of the four participating European Cities, using them each two days period COVID-19 measure values and each pollutant gas density. Figures show that there is no measure with strong correlation, thus they used all the measures for the machine and deep learning models. The machine learning outputs were sufficient enough for future predictions of the densities for each pollutant gas in a two days period. However, using a bigger dataset the results can be further improved. Models like these can be used, in the near future, for the estimation of the benefits from the replacement of petrol and oil vehicles, with other environmentally-friendly vehicles.